In today’s rapidly evolving business landscape, establishing robust GenAI and machine learning capabilities is of the utmost importance, especially for enterprises managing substantial data volumes. Financial institutions, notably banks, grapple with an immense wealth of data encompassing customer demographics, transaction records, and interaction data. The imperative task at hand involves facilitating effortless data accessibility, proficient organization, and harnessing this data to understand every customer at the N=1 level and enhance the customer experience.
Come along with me as we discover how a chance encounter during an informal meeting led to a game-changing improvement in a bank’s ability to leverage Generative AI and machine learning for hyper-personalized banking.
During my recent trip to London for a conference focused on how big data influences customer experience in financial institutions, I had an intriguing encounter. Post an insightful day, while enjoying the evening refreshments, I met Natalia, a high-ranking officer in the retail banking division of a prominent regional bank.
Natalia’s Dilemma of AI/ML in Banking
Our conversation quickly turned to Natalia’s challenges in boosting revenue. She opened about the evolving trends in the banking sector and its digital transformation. Natalia expressed a mix of optimism and skepticism regarding the use of Generative AI in banking. Her enthusiasm for this disruptive technology was tempered by concerns about time to market and their practical implementation and efficiency.
The core of Natalia’s doubts lay in her recent experience with a machine learning solution sourced from an external vendor. Despite the advanced technology, her team struggled to effectively target potential customers to cross sell the bank’s products. A significant gap existed between the banking team’s on-the-ground knowledge and the vendor’s machine learning expertise. This disconnect meant that the bank’s deep understanding of its customer base was not being leveraged to its full potential. The solution provided by the vendor was somewhat of a ‘black box’—opaque and difficult to consume or modify.
While listening to her story, I had a smile on my face as I knew that I had the perfect solution for her. I did not say anything then but requested a meeting in her office the next week to learn more about the problem.
Decoding the bank’s challenges and solutions
On my visit to Natalia’s office, she introduced me to Tereza, the CVM Head of Retail at the bank. Tereza was working on building a machine learning model for Next Best Product to offer to a customer by leveraging customer interaction data and transaction data for improving customer experience and personalized offers. Based on my conversation with Tereza and the time I spent with her, I gained a fair understanding of solving this business problem and the challenges she faced:
Data Integration and Quality
Tereza struggled with consolidating diverse data sources, such as transaction histories, customer interactions, and latest KYC data. Ensuring the accuracy, completeness and quality of this data is crucial but often challenging, as it involves cleaning, standardizing, and merging data from various systems.
Tereza needs an interface/platform that allows her to connect to different data sources and have a singular view. The platform also has the capability to pre-process the data, feature engineer, basically get data ready for modeling.
Privacy and Compliance
With the increasing emphasis on data privacy and protection, Tereza must ensure that the bank’s use of customer data complies with regulations. This involves careful handling and processing of personal data while maintaining customer trust.
Tereza will be most satisfied with a solution which is available on-premises for maintaining all the privacy overhead and also ensuring full visibility across the whole machine learning lifecycle.
Enterprise-wide Collaboration
Tereza collaborates with several stakeholders to build this solution. She needs help from analysts and the business team for some domain expertise to build an accurate approach for the solution. She asks the IT team to connect to relevant data sources and help her with required data extraction. She needs to make sure the data engineering/scientist team validates the data and has the required infrastructure to start the modeling process.
Tereza and her colleagues need a solution to smoothly collaborate for all stakeholders to have access to complete processes at all stages, increasing the pace and efficiency of collaboration.
Advanced Analytics Capability and Ease of Use
Developing a sophisticated machine learning solution for ‘Next Best Product’ recommendations requires advanced analytics capabilities. Tereza must ensure the solution is not only accurate but also adaptable to changing customer behaviors and market trends. However, this often demands deep technical expertise, which can be a barrier for teams with varying skill levels.
A platform that simplifies the creation and management of complex analytics models, useful even to those with less technical expertise, would be invaluable. It should offer a low code-no code, user-friendly interface that allows Tereza and her team to easily build, test, and iterate models without needing in-depth coding skills.
Scalability and leveraging full potential of Modeling Results
As more machine learning model use cases are tapped in, the infrastructure supporting it must be able to scale linearly as needed. Also, all the necessary stakeholders should be able to consume valuable insights in a seamless fashion. Tereza faces the challenge of ensuring that the system remains efficient and responsive, even as the amount of data and the complexity increase.
A solution that not only scales effectively but also allows easy consumption of modeling results in plain language where senior management can talk to their data to gain non-obvious valuable insights.
In a nutshell, my interactions with Natalia and Tereza revealed a sector at the cusp of a digital transformation yet hindered by technical and practical challenges. It underscored the need for solutions that are not only technically proficient but also intuitive, compliant, and collaborative in nature. As I concluded my visit, I was more convinced than ever of the value LumYn could bring to their institution, promising a blend of innovation and practicality in the dynamic world of banking.
LumYn is a GenAI-enabled growth intelligence platform that leverages data and machine learning to understand each customer at N=1 level and make predictive decisions to determine the next best action for further engagement.
- Conversational AI: Enables business users to interact with customer data (transactions, behaviour, and interactions) using natural language, facilitating seamless insight generation and growth strategy development.
- Rapid Development: Customizable AI models tackle unique business challenges, promoting growth. The low-code platform allows for quick iteration and refinement, ensuring rapid adaptability.
- Maximized Customer Lifetime Value: Strengthens customer relationships by creating behavioural segments based on spending habits and lifestyles, enabling targeted marketing campaigns.
- Accelerated Time-to-Market: Leverages a comprehensive library of pre-built AI models, delivering faster insights and quicker deployment, enhancing cross-sell opportunities.
- Reliable and Secure AI: Ensures complete data transparency with visible modelling processes. Built on robust technologies that prioritize security and privacy, LumYn offers a trustworthy AI solution for banks.
You might be interested in
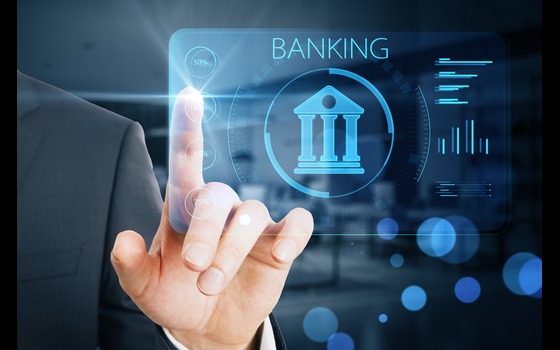
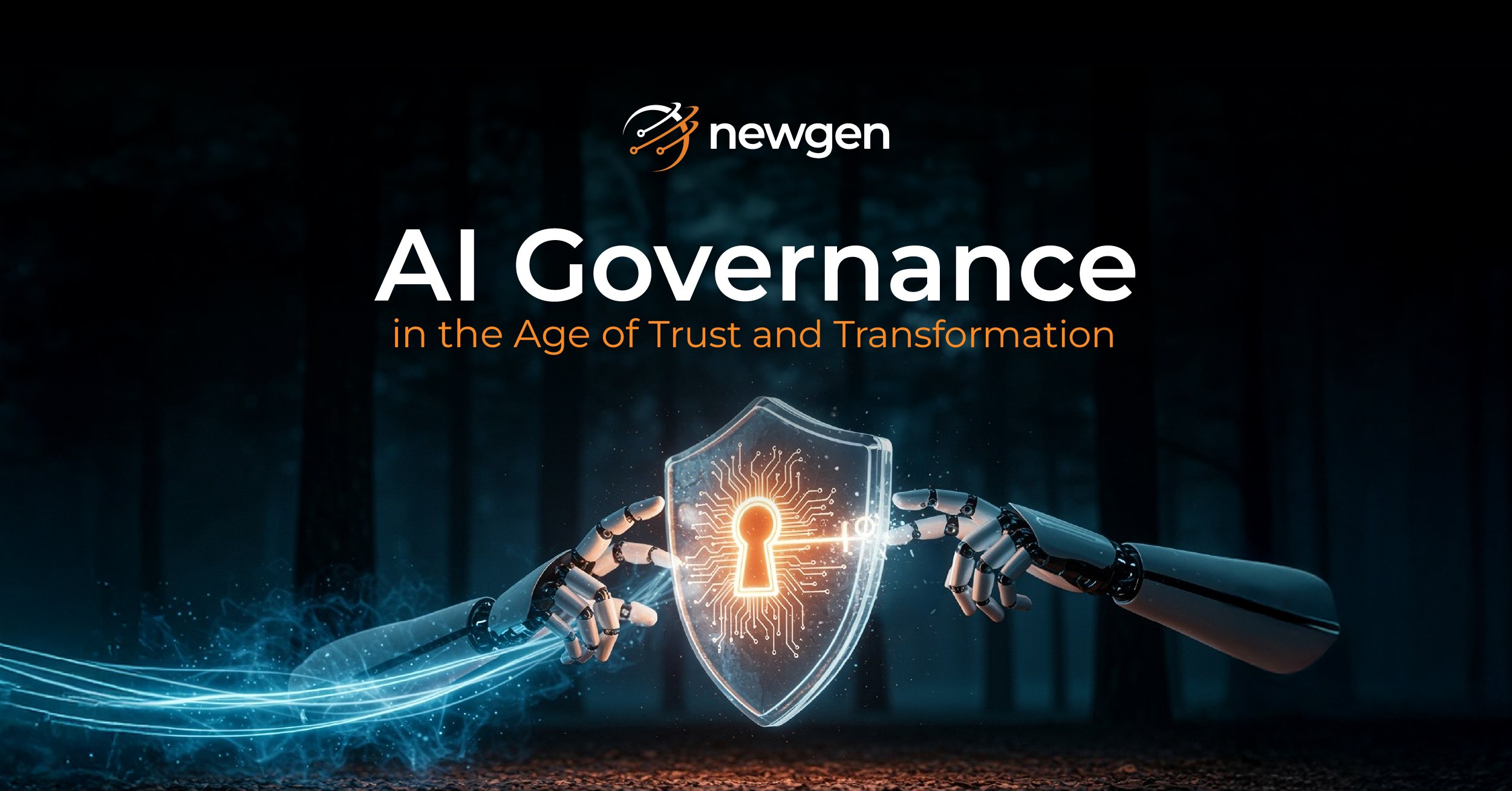
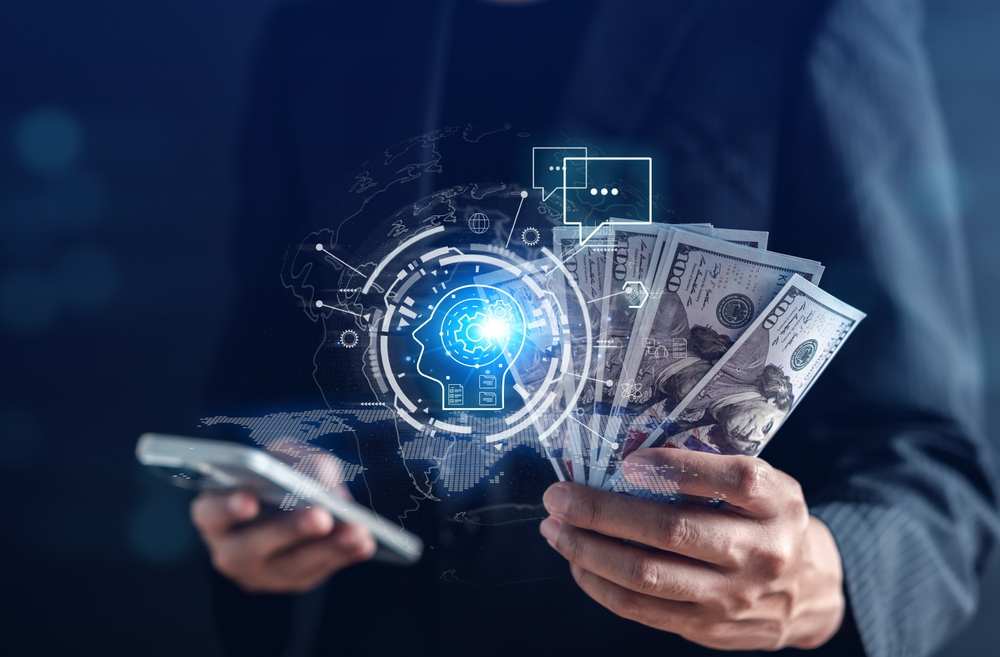