Did you know that the Artificial intelligence market has grown by 20% every year for the past five years? Given these rising figures and the benefits it offers, businesses and organizations have been seeking ways to employ AI more efficiently in managing and processing vast amounts of information related to their enterprise.
Research shows that businesses spend more than $30 million to extract data from documents manually. Gartner reports that incorporating intelligent data extraction will help employees quickly skim through the data and translate it instantly into simplified insights.
A next-gen technology, intelligent data extraction helps in transforming raw data into usable formats, delivering valuable insights for business operations. Want to know more? Scroll down!
What is Intelligent Data Extraction?
Intelligent data extraction is the process of automating the extraction of valuable information from various types of data sources. The technique leverages a combination of AI techniques to ensure the accuracy of extracted data.
Intelligent data extraction systems are designed to understand the context and semantics of both structured and unstructured data, making them more accurate and flexible than conventional data extraction methods.
Below are some crucial components of AI underlying intelligent data extraction that revolutionize the business process.
1. Natural Language Processing (NLP)
NLP is a branch of AI that employs complex algorithms to help computers recognize, interpret, and generate languages. It assists in classifying data, understanding language context, translation, intelligent data processing, and information extraction.
2. Large Language Models (LLMs)
LLMs are a framework that is a subset of NLP. They are systems trained on a substantial dataset of text to develop an understanding of patterns in language and logic. Neural networks (transformers) bridge the gap between the massive data in LLMs and the algorithms that empower computers to build articulation communication and better insights. This crucial aspect of AI is used to develop intelligent data insights from your contract metrics.
3. Machine Learning (ML)
ML utilizes all of the functional features of AI algorithms to learn without additional programming to analyze patterns and compare data quality and accuracy. It sometimes uses human-assisted training to build autonomous comprehension. Machine learning is majorly used for image recognition in data scanning and extraction and in guiding through classifying content.
How does Intelligent Data Extraction work?
When you have the proper knowledge, you can easily employ intelligent data extraction solutions in our workflow. The rise in its global market, which stood at USD 1.45 billion in 2022 and is projected to rise at a CAGR of 30.1% between 2023 and 2030, is a testament to its potential. Let’s explore how the system works.
Step 1 – Data Ingestion
Every process for intelligent data extraction begins with raw material—the influx of raw data into your system. You should collect every type of document from various sources. These diverse data points, when ingested collectively, will set the foundation for the forthcoming process.
Step 2 – Pre-processing
Data pre-processing ensures that you are using only relevant, clean data for the process. Unwanted elements will be filtered out to ensure consistency. The following processes will happen in this step.
- De-skew – It corrects any national misaligned in scanned or captured images to make sure that the text or content is horizontally aligned for accurate processing.
- Binarization – It is a basic image processing technique that converts a grayscale image into a binary image where each pixel is classified as either black or white based on the defined threshold.
- Zoning – In this process, the image will be divided into zones or regions. The segmentation will allow the intelligent data extraction system to focus on the specific areas of interest within the document for better accuracy and efficiency.
- Normalization – It involves standardizing the image quality and size to best fit. It includes adjusting contrast, brightness, and resolution to enhance the clarity of the content, making it easier for further steps.
Step 3 – Training the Algorithm
In this phase, machine learning models will be trained to use sample data, making them recognize patterns and relationships. They will be trained using thousands of images or documents, helping to improve the standing of falsified fat files like name, income, employment history, and more.
Step 4 – Data Extraction
In this step, the system will retrieve the pertinent data that points to the business. It involves particular specifics like personal details from a form or amounts from a bank’s transaction history. The trained algorithm will now sift through massive datasets and extract vital details with an improved accuracy rate.
Step 5 – Data Validation
Validation is a simple quality-check process to ensure the data extraction is performed flawlessly. A validation step will cross-check the email details with predefined rules or reference datasets.
Step 6 – Continuous improvement
The world of data is evolving, and so should the algorithms of intelligent data extraction. They should continuously learn from the data to refine their accuracy. As your business rolls out new services, products, or changes in the structures, the extraction algorithm will adapt, ensuring it is relevant and reliable every time.
How Intelligent Data Extraction Complements OCR?
OCR is also sometimes referred to as text recognition. An OCR program extracts and repurposes data from scanned documents, camera images, and image-only PDFs. It singles out every letter in the image, converts them into words, puts the words into sentences, and provides access to edit the original document.
This technology eliminates the need for manually entering the data. Studies have shown that documentation affects productivity, and around 46% of employees spend their time completing inefficient paper-based tasks. That’s why many businesses have adopted intelligent data extraction to streamline all document-related tasks.
This can be used to complement the OCR systems. Cutting-edge technologies are being developed based on computer vision in ML (machine learning) and NLP (natural language processing) to execute the extraction of useful information from the images of scanned documents.
Intelligent Data Extraction relies on Optical Character Recognition (OCR) and Natural Language Processing (NLP). Let’s see how:
- OCR: OCR converts the text in the images into machine-readable format (machine-encoded text). This extraction goes in-hand with other methods, like computer vision (box and line detection to extract tables and key-value pairs).
- NLP: NLP is required to analyze the meaning of the converted text.
So, OCR software deciphers the text in the images or scanned documents. This process can be automated, and it is called OCR data capture. The enterprise system includes interfaces for document recognition, scanning, data verification, and export. Besides, the automation also offers workflow management and monitoring capabilities to track huge amounts of data.
The OCR data capture process, which includes OCR and data extraction, is the process of transforming a document into live data ready to be used for further processing. Here’s how this process works:
Step 1.
Choose appropriate data to extract. It is usually challenging if the source documentation is poorly formatted, and this is where automated metadata management can assist. You can import the data, annotate it, and create an execution plan separate from the transaction processing program.
Step 2.
The main focus is on the image quality. The OCR engine automatically checks for mistakes and corrects them.
Step 3.
Now, the format of the scanned documents (like JPG and PNG) and their structure (like structured, unstructured, or semi-structured) will be identified.
Step 4.
The document will be divided into sections, sub-sections, tables, or zones. The critical characters will be identified.
Step 5.
Any inaccuracies or errors will be identified to increase the data accuracy.
Step 6.
All the flagged documents will be examined for the most precise data extraction model. The software pushes the extracted and cleaned data to the OCR database, or it can export it in a variety of formats after that.
The OCR and data extraction process gives an appropriate methodology to access data which is kept in different formats. This data can be utilized for various purposes, including marketing, research, and decision-making.
Benefits of Intelligent Data Extraction
Implementing intelligent data extraction in your business’ infrastructure can transform the way you handle data. Here are some key benefits.
1. Better scalability
Intelligent data extraction technology is highly scalable and suitable for businesses of all verticals and sizes. Whether your business needs to process a few hundred documents a month or thousands of documents in a day, the solutions can be tailor-made to meet your requirements.
2. Improves organizational synergy
Intelligent data extraction serves as a cohesive force within a business that promotes collaboration and knowledge-sharing. When employees are free of handling manual data entry and extraction tasks, they can direct their focus on strategic and creative endeavors. This will improve synergy and lead to better cross-functional cooperation, allowing your business to access accurate and up-to-date data.
3. Cost-effective
Intelligent data extraction technique is not just a time-saver but also a cost-effective solution. Automating the data extraction process can reduce the operational costs associated with manual data entry errors or any time delays. For instance, in supply chain management, the technology will streamline the process of invoices, purchase orders, and shipping documents. It will automate the upcoming time and minimize any expensive errors.
4. Seamless data management
Intelligent data extraction techniques often deal with data from diverse origins, with unique formatting and structure. They facilitate the easy retrieval and analysis of such data, supporting better decision-making and operational efficiency of your business.
5. Improved compliance
Many businesses are subject to stringent regulatory requirements regarding data handling and reporting. Intelligent data extraction helps you to ensure that the data is processed consistently and accurately, making sure to comply with the respective regulations.
Summing Up!
Nowadays businesses have access to sustainable data resources, both structured and unstructured. The vast data comes with the potential to unlock valuable insights, which will improve your decision-making and drive innovation. Employing the right intelligent data extraction will transform your business and open doors to streamlined processes, giving you a competitive edge in the digital age.
Newgen’s Intelligent Data Extraction helps you unlock the potential of AI for your business and take the next step toward innovation. Our strategic collaboration will help you navigate the competitive business landscape, and analyze data from varied sources sustainably that will maximize your productivity with minimal manual intervention.
Frequently Asked Questions (FAQs)
Q1. What is smart data extraction?
A smart data extraction refers to a sophisticated, best-in-class software tool that equips advanced algorithms and machine learning techniques to automatically extract relevant data and information from various data sources across the digital space. Intelligent data extraction is one such smart solution that seamlessly identifies and extracts key data for smooth data analysis.
Q2. What is intelligent data processing?
Intelligent data processing refers to the act of using advanced Artificial intelligence algorithms and machine learning techniques to analyze and understand semi-structured and unstructured data, regardless of the format or medium. This data can include text, audio, video files, social media posts, emails, and any other form of data source.
Q3. What is the difference between IDP and OCR?
Both IDP (Intelligent Data Processing) and OCP extracted text from images and scanned documents to save time and make informed business decisions. OCP is a customary technique that uses predefined, customized templates to run processes that are prone to errors. However, IDP uses the latest AI technology to be much more accurate than OCR and can steadily extract text from various documents.
Subscribe to our blog
You might be interested in
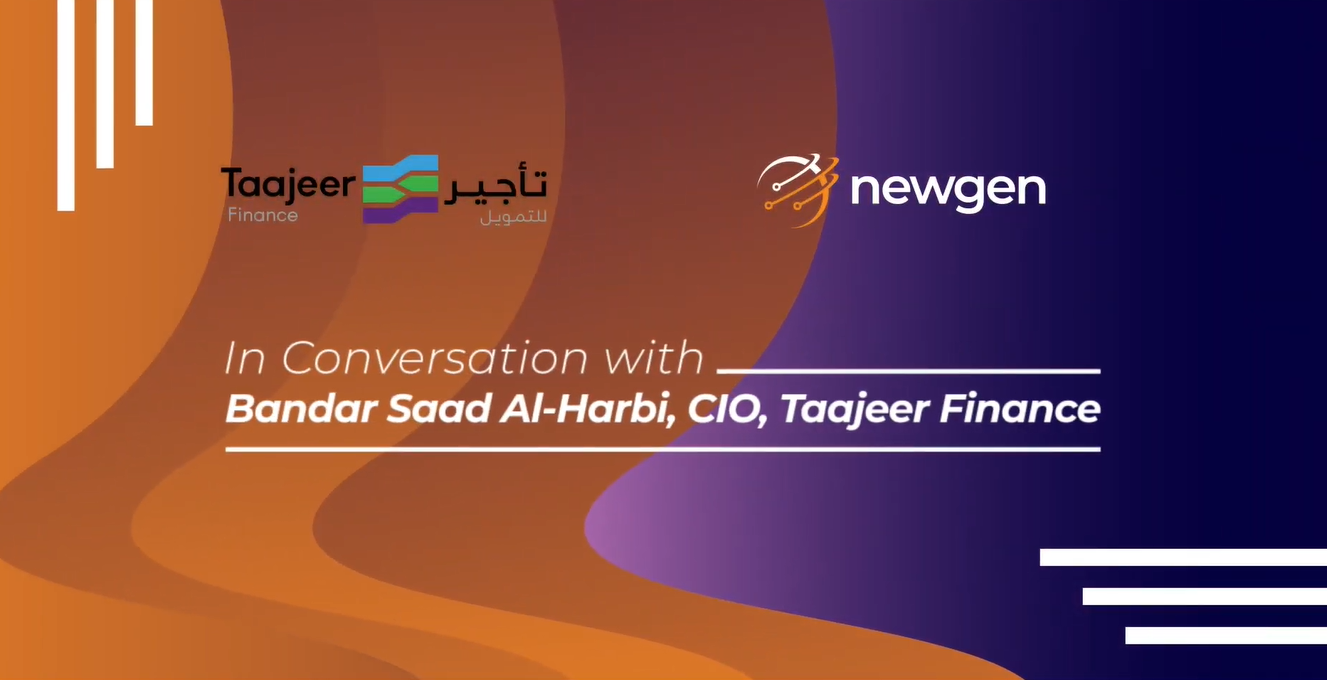
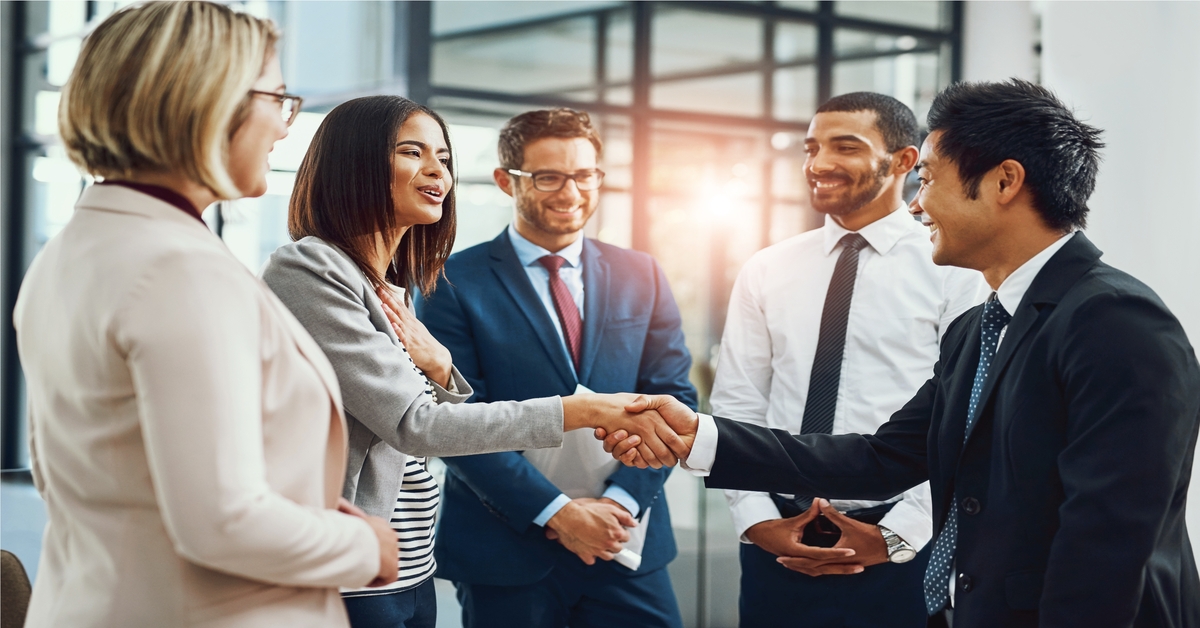
24 Jul, 2024
Case Study: One of the Largest Banks in the UAE Streamlines Onboarding and Lending Process with Newgen
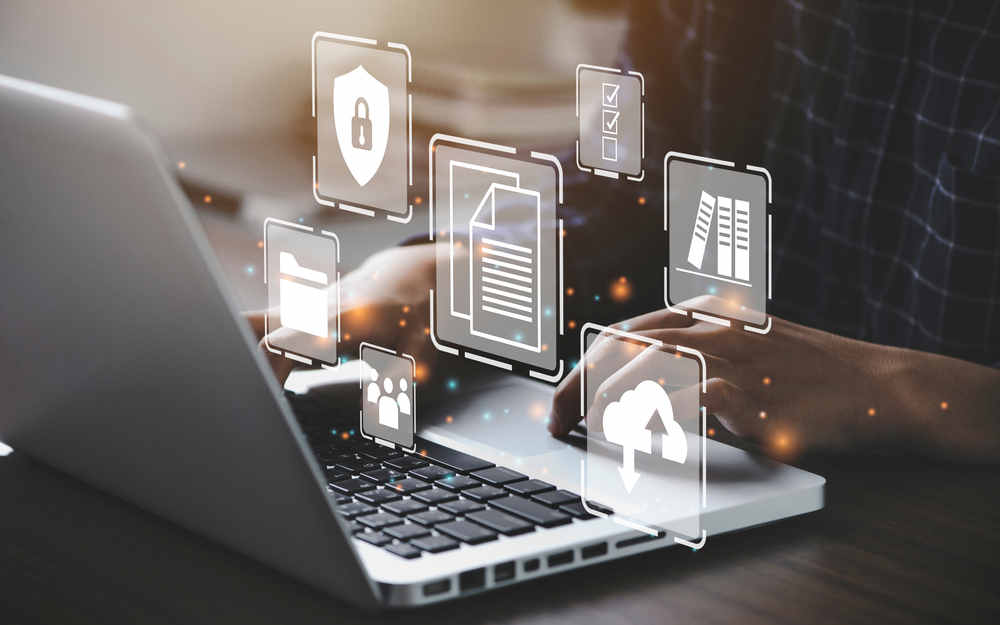
23 Jul, 2024
Case Study: One of the Top International Energy Alliances Streamlines Document Management with Newgen