Artificial intelligence (AI) has become a part of our everyday life. If you think about it, these experiences may feel common to you:
- Shopping app recommending the perfect T-shirt to match your trousers
- Bank sending a fraud alert when something looks off in your account
- Music app curating a playlist to perfectly match your vibe
Ever wondered, what makes these smart, seamless experiences possible?
The answer is AI workflows.
What is an AI Workflow?
An AI workflow is a step-by-step process that helps businesses turn data into smart decisions. It uses AI to collect, clean, and analyze data, and then delivers insights or acts on it.
With AI workflows, businesses can:
- Automate task
- Make accurate predictions
- Offer personalized experiences
- Improve efficiency and reduce operational cost
How Do AI Workflows Work?
It’s like a journey of your data—from raw to ready.
Here’s a simple breakdown:
- Data is collected from different sources
- It’s cleaned and prepared for use
- AI models are trained using this data
- The model is tested, then deployed
- The system is monitored to ensure ongoing accuracy
- Each of these steps is supported by specific AI technologies
Key Components of AI Workflow and How it Works
1. Application Programming Interface (API) & Optical Character Recognition (OCR)
The journey begins by gathering data from diverse sources – be it customer records, sales reports, or cheque readings. APIs pull this information from platforms like CRMs or websites. OCR steps in to extract text from scanned files. Once the information is gathered, the data is cleaned and structured.
2. Natural Language Processing (NLP)
This is the learning phase. Clean data is fed into the system, where machine learning (ML) algorithms help the AI detect patterns and make informed predictions. If the data involves language, NLP comes into play, enabling the AI to understand human inputs, including emails, chats, or feedback and respond intelligently is another process.
3. Machine Learning (ML)
Before an AI model is deployed, it goes through an evaluation of accuracy and reliability. Here, ML techniques play a key role in measuring its performance, fine-tuning the results, and making sure AI is ready to handle real-world situations efficiently.
4. Intelligent Process Automation
Once the model is fully trained, it’s integrated into the actual ecosystem – automating tasks, analyzing live data, or delivering insights that guide decision-making. Tools such as intelligent automation handles more complex tasks, such as scheduling or dynamic pricing, and business process automation (BPA) ensures repetitive tasks, like processing orders or managing customer queries, run smoothly without human intervention.
5. Generative AI (GenAI)
AI evolves with data. Continuous monitoring ensures the system stays error-free and relevant. ML algorithms help models adapt over time, while GenAI creates fresh insights or generates content based on the latest data – helping businesses stay ahead of the trend.
Common Use Cases of AI Workflows
AI is creating value beyond the hype by shifting from experimentation to real-world applications. Here are some of the most common and high impact use cases:
1. Customer Service: Chatbots and Virtual Assistants
Gone are the days of endless hold music. Chatbots and virtual assistants are now handling everything from booking appointments to resolving basic complaints — and they’re getting better at it every day. These tools learn from each interaction, improving response time and creating a smoother, more satisfying customer experience.
2. Healthcare: Early Detection with Predictive Diagnostics and Medical Imaging
In medicine, speed and accuracy can be lifesaving. AI can help doctors spot early signs of serious conditions like cancer and stroke by analyzing CT scans, MRIs, and other imaging data. These systems don’t just read images — they assist in diagnosing, flagging issues faster and often with more precision than traditional methods. The result? Better care and improved outcomes for patients.
3. Finance: Fraud Detection and Credit Scoring
Banks and financial institutions are using AI to fight fraud in real time. These systems monitor transactions as they happen, spotting unusual patterns and flagging potential threats instantly — long before they affect customers. It’s a smart, scalable way to protect both institutions and the people who rely on them.
4. Supply Chain & Logistics: Demand Forecasting and Route Optimization
AI workflows in these organizations use historical data, weather conditions, traffic patterns, and delivery schedules to forecast shipment volumes and determine the fastest, most fuel-efficient delivery routes. AI systems continuously adjust predictions and operations based on real-time data, reducing delays and optimizing resources.
Benefits of AI Workflows
A report from the IBM Institute for Business Value reveals that 92% of executives believe their organization’s workflows will be fully digitized and driven by AI-powered automation by 2025.
Furthermore, the workflow automation market is expected to witness a CAGR of 5.8% over the forecast period, 2021-2026.
This highlights how organizations are quickly adapting to AI workflow to expedite their operations and improve overall business performance. Here are some key advantages:
1. Efficiency and Automation
- Automates repetitive and time-consuming tasks to reduce manual efforts. AI models can process large volumes of data quickly and provide insights or predictions, expediting decision-making processes
2. Consistency and Accuracy
- Ensures consistent follow up of processes to reduce human error. Workflows help manage model versioning, testing, and validation
3. Collaboration and Transparency
- Makes it easier for teams to understand, audit, and refine AI processes. Additionally, it also logs and monitors every step in the AI lifecycle for accountability
4. Cost Reduction
- Identifies inefficiencies and ensures lesser manual intervention, saving time and labor cost
Best Practices for Building Effective AI Workflows
To get the most out of your AI workflows, consider these practical strategies:
- Start with a Clear Objective: Know what business problem you’re solving and align your workflow to address it
- Use High-quality, Diverse Data: Clean, relevant, and representative data improves model accuracy and workflow output
- Modular Architecture: Break the workflow into manageable components (e.g., data ingestion, training, and deployment). This makes it easier to troubleshoot and scale
- Human-in-the-loop (HITL): Incorporate checkpoints where human review enhances model performance or handles exceptions
- Regular Monitoring and Feedback Loops: Continuously assess and refine workflows using performance metrics to keep the models current and effective
- Security and Compliance: Ensure data privacy, especially in regulated industries like healthcare and finance
Future Trends in AI Workflows
AI workflows are rapidly evolving. One major shift is the integration of MLOps, a fusion of machine learning and DevOps, that helps streamline model deployment, monitoring, and scalability across ecosystems.
As speed becomes critical, Edge AI workflows are gaining momentum by enabling models to run closer to the data source, such as on devices or sensors, ensuring faster and real-time decision-making.
Summing Up
Behind every smart recommendation, real-time alert, or seamless experience lies an invisible engine – AI workflows. They are not just tools but enablers of smarter, faster, and more human-centric outcomes. As businesses move from automation to autonomy, mastering AI workflows becomes less about catching up with the trend and more about staying ahead.
You might be interested in
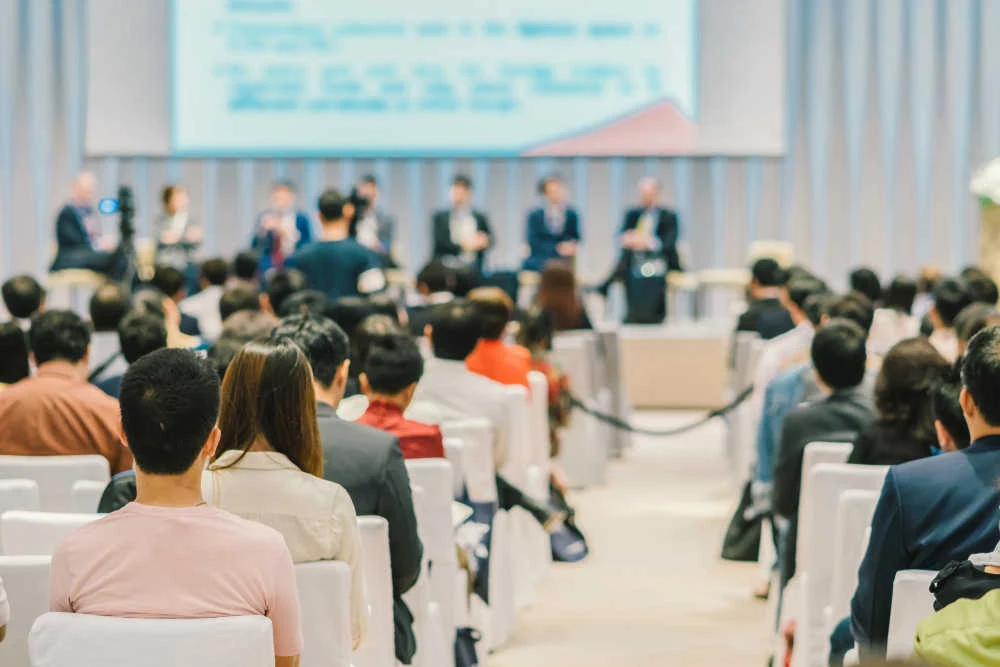
15 Jan, 2025
2025 and Beyond: How AI is Reshaping Digital Lending in Banking & Financial Services