In recent months, the term “Generative AI” has apparently become more popular in digital times. There has been a noticeable increase in interest, according to Google Trends. The introduction of generative models such as DALL-E 2, Imagen, and ChatGPT is likely the reason for this interest. Within the tech industry, generative artificial intelligence (AI) has become a disruptive tool. However, what exactly is it? And why is it becoming so popular? Also, as artificial intelligence (AI) develops, so do concerns regarding its use and advancement.
Generative AI raises issues with data bias, intellectual property rights, and potential misuse or manipulation. In order to ensure that generative AI technologies are developed and used responsibly without damaging people or society at large, it is essential to comprehend and address these concerns with potential strategies. The article will examine the generative AI definition, along with its applications and best practices.
What is Generative AI?
Generative AI, such as deep learning models, can generate highly realistic text, images, and other data based on the input data with which they were trained. This technology is fast gaining momentum, with many creative applications across varying industries.
Personalized treatments are accelerating and improving healthcare services, while adaptive learning is revolutionizing educational methods. These are just a few generative AI examples of how it is transforming content creation in media and marketing.
How Does Generative AI Work?
A prompt is the first step in the generative AI tool working process. This prompt can be text, an image, a video, a design, musical notes, or any other type of input that the AI system is capable of handling. Then, in response to the prompt, different AI algorithms return fresh content. Content can be essays, problem-solving techniques, or real imitations made from images or voice recordings of real people.
In the early days of generative AI, data submission required the use of an API or other challenging procedures. The developers learned how to use specialized tools and write programs in advanced languages like Python.
The user interface and model of generative AI platforms are simple, user-friendly, and robust, so users can describe a request in simple terms. After receiving an initial response, you can further customize the outcomes by providing input regarding the tone, style, and other aspects you would like the generated content to encompass.
Generative AI Models
Generative AI models represent and process content by combining different AI algorithms. To produce text, for example, different natural language processing methods convert raw characters (such as letters, punctuation, and words) into sentences, entities, and actions. These are then represented as vectors using a variety of encoding techniques. Similarly, vectors are used to express different visual elements from images.
Developers use a specific neural network to create new content in response to a prompt or query once they have decided to represent the world. Neural networks with a decoder and an encoder, or variational autoencoders (VAEs) and Generative adversarial networks (GANs) models, are among the techniques that can be used to create AI training data, realistic human faces or even individualized human idols.
Recent developments in transformers, like Google’s Bidirectional Encoder Representations from Transformers (BERT), OpenAI’s GPT, and Google AlphaFold, have also led to the development of neural networks that are capable of producing new content in addition to encoding language, images, and proteins.
What are the Concerns Surrounding Generative AI?
The rise of generative AI is also raising several concerns. The quality of the results, the possibility of abuse and misuse, and the potential to upend current business models are all related to these concerns.
Here are a few examples of the specific types of challenging issues that the state of generative AI now represents:
- It may offer false and deceptive information.
- It is more difficult to trust someone if you don’t know where they got their information.
- It may encourage novel forms of plagiarism that neglect the rights of original content creators and artists.
- It might disrupt current business models that rely on advertising and search engine optimization.
- It facilitates the production of false news.
- It makes it easier to argue that genuine photographic evidence of wrongdoing was merely an AI-generated fake.
- It might pose as a person to carry out more successful social engineering cyberattacks.
Enterprises should adopt sound AI engineering practices and make responsible AI a cornerstone of their GenAI efforts in order to prepare for the inevitable “trough of disillusionment” that comes with emerging technology, given the novelty of Generative AI tools and their rapid adoption.
Use Cases for Generative AI by Industry
The broad range of applications and industries that new generative AI technologies can impact has led to comparisons with general-purpose technologies such as electricity, computing, and steam power. Remember that while new approaches can speed up small portions of existing workflows, it often took decades for people to figure out how to organize workflows best to take advantage of them, similar to the earlier general-purpose technologies. Let’s understand how Generative AI application boosts productivity in different industries:
- Finance can monitor transactions in relation to an individual’s past in order to improve fraud detection mechanisms.
- The technologies under generative AI could help law firms draft and interpret contracts, infer evidence, and develop arguments.
- Generative AI models combine information from cameras, X-rays, and other metrics so that manufacturers can pinpoint the defective part and find the root cause far more precisely and cost-effectively.
- Generative AI can help media and film companies create content more affordably and translate it into other languages using the actors’ voices.
- It can analyze complex genetic data and molecular data to create personalized treatment plans for patients.
- It can assist architectural firms create and modify prototypes more quickly.
- Gaming companies can use generative AI to create game levels and content.
Generative AI vs. AI
Naturally, generative AI shares many attributes with traditional AI. But there are also some stark distinctions between Generative AI and AI.
Aspects | Gen AI | AI |
---|---|---|
Generative Models | Generative AI uses neural network techniques like transformers, GANS, and VAEs. | Other types of AI include reinforcement learning, recurrent neural networks, and convolutional neural networks as their methods for realizing their functions. |
Focus Output | It generates unique data by utilizing human input and analytical data. You could think of this AI as a creative assistant. | Its application is limited. The model performs certain tasks, finds patterns, and makes predictions by analyzing data. It is an incredibly effective problem solver. |
Applications | It is used in content creation processes, including image generation, music composition, and writing. | Traditional AI is most notably applied in fraud detection, spam filtering, and recommendation systems. |
Transparency | It can be less transparent due to the complexity of its learning algorithms, making it difficult to understand how it appears at specific outputs. | It frequently implements predetermined rules, which facilitates a more transparent and understandable decision-making process. |
Best Practices for Using Generative AI
Depending on the modalities, workflow, and intended goals, there are different best practices for applying generative AI. However, when using generative AI, it’s critical to take into account important elements like accuracy, transparency, and usability. The following procedures help in achieving these elements:
- All generative AI content should have clear labeling for consumers and users.
- Verify the content’s accuracy using primary sources when needed.
- Understand how bias might be woven into the AI results.
- Use additional tools to verify the accuracy of AI-generated content and code.
- Discover the benefits and drawbacks of every generative AI tool.
- Introduce yourself to common failure ways in results and devise strategies to prevent them.
Utilizing the power of generative AI, redefine how your employees work by increasing productivity and establishing countless opportunities. With NewgenOne Marvin, you can design and develop business apps more quickly, get real-time insights from your documents and processes, and create meaningful customer interactions.
To help you quickly achieve end-to-end automation, we’ve combined content management, customer engagement and process automation in an advanced low-code platform called Newgen ONE. Imagine now how generative AI might increase it even more! Here are the features of our NewgenONE Marvin:
- Design and build applications superfast
- Get even more out of content with automatic categorization
- Get creative and personalize communication faster
- Go visual with intelligent insights
Bottom Line
As part of augmented analytics workflows, Generative AI could help with data processing, transformation, labeling, and vetting. Generative AI can be used by semantic web applications to map internal taxonomies describing job skills to various taxonomies on sites that provide skills training and recruitment. Business teams will also use these models to label and transform data from third parties in order to perform more advanced risk assessments and opportunity analyses.
In the future, generative AI models will support supply chains, business processes, drug development, digital twins, 3D modeling, and product design. As a result, it will be simpler to develop new product concepts, test various organizational structures, and investigate different business ideas.
Frequently Asked Questions
What are some gen AI tools?
Generative AI tools are available for a number of modalities, including text, images, music, code, and voices. The following are a few popular AI content creators to look into:
- GPT, Jasper, AI-Writer, and Lex are examples of next-generation tools.
- Dall-E 2, stable diffusion, and Midjourney are a few image-generating tools.
- CodeStarter, Codex, GitHub Copilot, and Tabnine are a few examples of code generation tools.
What are the limitations of Gen AI?
The following are some limitations to take into account when developing or utilizing a generative AI application:
- It does not always determine the source of the content.
- It can be difficult to determine the bias of sources.
- Content that sounds realistic can make it more difficult to identify inaccurate data.
- It can be challenging to figure out how to adjust to new circumstances.
- The results can hide bias, prejudice, and hatred.
What is the future of Gen AI?
The remarkable complexity and user-friendliness of ChatGPT encouraged generative AI to become widely used. The field of generative AI will continue to evolve, leading to advances in translation, medication discovery, anomaly detection, and the creation of original text, video, music, and fashion design.
What are the benefits of Gen AI?
The following are some potential benefits of applying generative AI:
- Automating the laborious process of creating content.
- Reducing the effort required to respond to emails.
- Improving response times for specific technical inquiries.
- Making realistic representations of people.
- Organizing complex information toward a coherent narrative.
- Streamlining the process of producing material in a specific manner.
You might be interested in
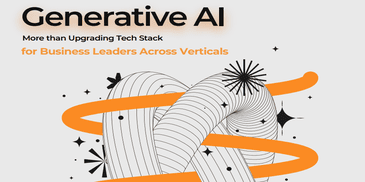
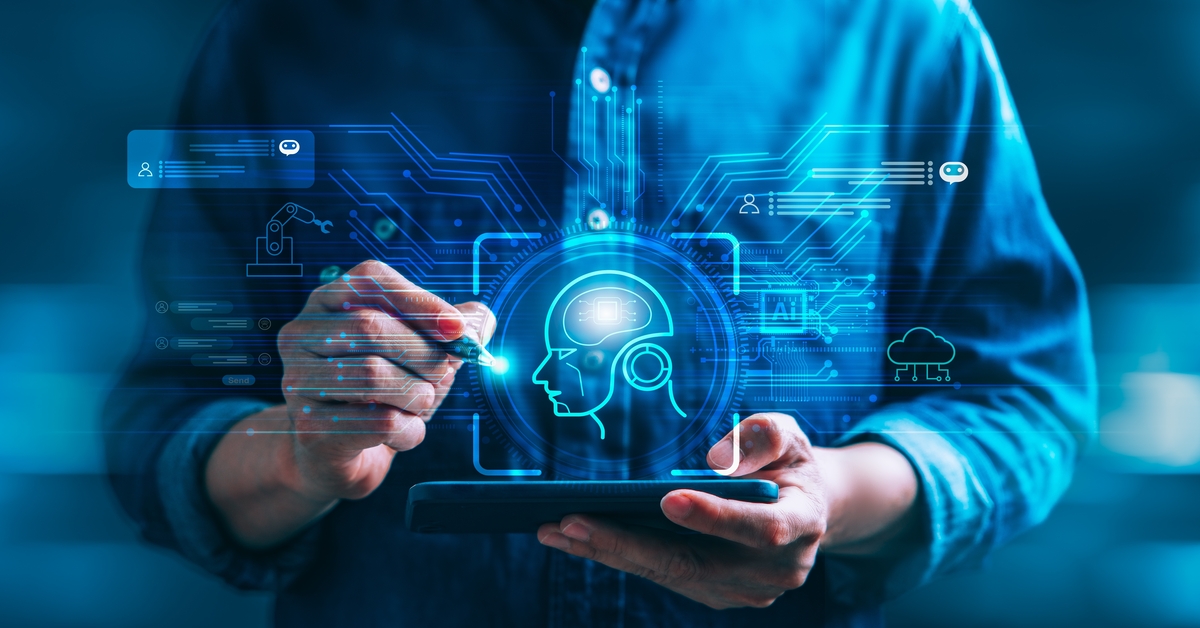
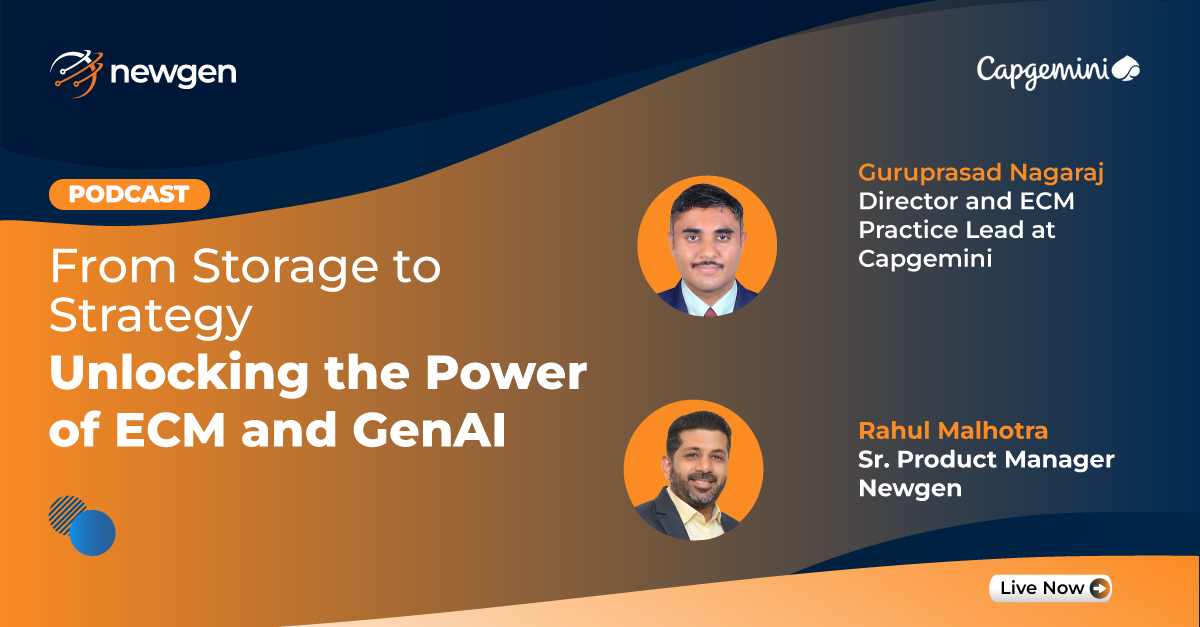